Can AI Responses Be Biased? Understanding Gender and Racial Prejudices in AI
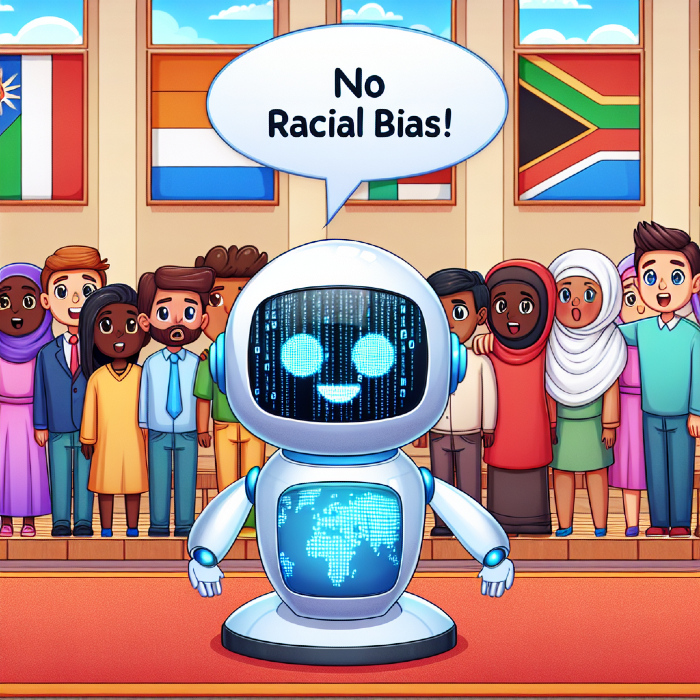
Artificial Intelligence (AI) has become a ubiquitous presence in our lives, from virtual assistants like Siri and Alexa to complex algorithms driving decisions in finance, healthcare, and law enforcement. However, as AI systems become more integrated into society, concerns about their potential biases have also grown. This blog delves into whether AI responses can be biased, specifically focusing on gender and racial prejudices, and provides examples to illustrate these issues.
Understanding AI Bias
AI bias occurs when an AI system reflects the prejudices present in the data it was trained on. These biases can manifest in various ways, often perpetuating existing stereotypes and inequalities.
Gender Bias in AI
Gender bias in AI is a significant concern. For instance, a study by MIT Media Lab found that facial recognition systems had higher error rates for women and people of color compared to white men. This discrepancy can lead to unfair treatment and reinforce gender stereotypes.
Example: Amazon's Recruiting Tool
Amazon developed an AI recruiting tool to automate the hiring process. However, the tool was found to be biased against women, as it favored male candidates for technical roles. This bias was attributed to the data the tool was trained on, which predominantly consisted of resumes from male candidates. Amazon eventually scrapped the project due to these biases.
Source: Harvard Advanced Leadership Initiative
Racial Bias in AI
Racial bias in AI can have severe implications, particularly in areas like law enforcement and healthcare. AI systems used in predictive policing, for example, have been criticized for disproportionately targeting minority communities.
Example: Predictive Policing
Predictive policing tools use historical crime data to predict where crimes are likely to occur. However, these systems have been found to reinforce existing racial biases in policing, leading to over-policing in minority neighborhoods. This can perpetuate a cycle of mistrust and discrimination.
Source: NPR
Addressing AI Bias
Addressing AI bias requires a multi-faceted approach, including:
- Diverse Data Sets: Ensuring that the data used to train AI systems is diverse and representative of all demographics.
- Regular Audits: Conducting regular audits of AI systems to identify and mitigate biases.
- Transparency: Making AI systems and their decision-making processes transparent to allow for accountability.
- Inclusive Development: Involving a diverse group of developers and stakeholders in the AI development process to bring different perspectives and reduce biases.
Case Study: Reducing Bias in AI
The American Civil Liberties Union (ACLU) has highlighted the need for greater oversight and regulation of AI systems to prevent discrimination. For example, AI tools used in housing have been found to perpetuate discriminatory practices, making it harder for people of color to find housing.
Source: ACLU
Conclusion
While AI has the potential to drive significant advancements, it is crucial to address and mitigate biases to ensure these technologies benefit all individuals equally. By understanding the root causes of AI bias and implementing strategies to counteract them, we can work towards creating fairer and more equitable AI systems.
By staying informed and proactive, we can help shape a future where AI serves as a tool for inclusivity rather than division.